Jindal School Researcher Suggests Strategy for More Efficient Data Acquisition
Jindal School Researcher Suggests Strategy for More Efficient Data Acquisition
By Glenda Vosburgh
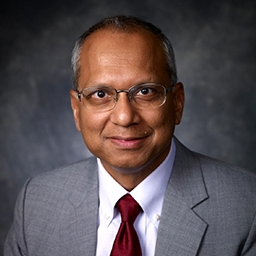
As the demand and cost of data acquisition grow, especially with vendors pricing data attributes differently, businesses face the challenge of identifying the most valuable data attributes to purchase within budgetary constraints.
A paper authored by a researcher at the Naveen Jindal School of Management identifies a solution that helps businesses solve that problem.
Published in the July 2023 issue of Management Science, one of the academic journals tracked in The UTD Top 100 Business School Research Rankings™, the paper — “Cost-Restricted Feature Selection for Data Acquisition” — was authored by Dr. Sumit Sarkar, the Charles and Nancy Davidson Chair, a professor in the Information Systems Area and director of PhD Programs at the Jindal School.
Customer data is an important tool for businesses and organizations that use it in analytics aimed at advancing operations, acquiring managerial insights and developing business strategies. New customer data is often used to initiate marketing campaigns to grow their customer base. The cost of the data owned by an organization is typically minimal when it is generated as a part of the company’s ongoing operations. When the company needs to acquire additional data for things such as promoting new products or expanding its customer base, the price of the additional data can be high.
“Data vendors have started pricing data at very fine granularities, often charging different amounts for each attribute of a record. There is a lot of data to consider and much of it will not be of value for a company,” Sarkar said. “Companies work within a budget and it is important that they be able to identify a set of the most useful and valuable attributes or features to acquire while keeping within their budget.” Companies that accurately identify the specific information they need can save money while obtaining the relevant data by purchasing only what is necessary.
The paper, which was also authored by Dr. Xiaobai (Bob) Li, a professor at the University of Massachusetts’ Lowell’s Manning School of Business; and Dr. Xiaoping Liu, an assistant teaching professor at Northeastern University’s D’Amore-McKim School of Business, presents a formula that businesses can use to identify the type of data they need to acquire. Once that is done, the business can tell the data supplier specifically what they need.
“If, for example, a bank wants to bring in new customers, it already has data about its existing clientele that it can use to help determine some of the attributes of its target market,” Sarkar said. The information it owns provides some information about potential new customers, but more information may be needed, such as a mailing list for prospective new customers. That information can be acquired from one of the many vendors that sell data in the current market.
“Using data it already has about existing customers, the company can develop a predictive model to determine what attributes for new customer prospects should be acquired,” Sarkar said.If the bank has three available attributes — age, gender and occupation — that are mostly equally important in identifying potential new customers, but the occupation data costs more than the age or gender data, budget constraints may dictate which two sets of data it acquires. The problem becomes very complex, Sarkar said, when many such attributes have to be evaluated.
“It is important to note that the solutions would be different for different firms,” he said. “For example, a hospital may need a different set of customer attributes than a bank, depending on cost structures and relevance to their marketing goals. The paper presents a methodology that each firm can adapt for their own use.
”The models described in Sarkar’s paper took about a year to develop and another year to fine-tune. They offer a new approach for “feature selection for customer data acquisition,” according to his paper, that is efficient and easy to use